Understanding advancements in AI and being able to separate facts from myths is important to understand.
Artificial Intelligence Research
In this article I would like to highlight the work of Ofosu-Ampong (2024), “Artificial Intelligence Research: A Review on Dominant Themes, Methods, Frameworks and Future Research Directions”.
The goal is to simply share some of the highlights of this work. I recommend that readers take the time to read the full paper. In this paper, Dr. Ofosu-Ampong shares some themes (explored further below) in current AI research. These categories of theme in AI research would be technological, contextual knowledge, conceptualization, and application domains. This article will explain these themes as noted by Ofosu-Ampong (2024). By illuminating contemporary AI challenges, methodologies in research, levels of analysis, conceptual strategies, and frameworks, Ofosu-Ampong notes that this study aids in the progression of AI research and identifies pathways for future investigation.
“Dr. Kingsley Ofosu-Ampong is a behavioral scientist at the Alliance of Bioversity International and International Center for Tropical Agriculture (CIAT) in Accra, Ghana. With a PhD in Information Systems and a degree in MBA and Sociology from the University of Ghana. His research interests include behavior change, information systems, sustainability, technology adoption and agronomic behaviors.” (p. 11)
The primary objective of this article is directed to our clients as well as “average” citizens who may not be well versed or up to speed on this crazy thing called Artificial Intelligence or all of its applications!
So, let’s explore AI a bit.
Article Contents
- We All See the News: AI is Here to Stay and Evolving Quickly!
- AI Uncertainty from Business and Individuals
- No Doubt About It: AI Will Bring Change
- We All Have a Role to Play in Determining the Future Role of AI
- How Can AI Research Help Address Future Uncertainty of AI Deployment?
- A Word on Research: Looking for Trends and Themes
- What is Artificial Intelligence (AI)?
- What Are Information Systems?
- Ofosu-Ampong, 2024: Dominant Themes
- Ofosu-Ampong’s Recommendations and AI Solutions for Businesses
- Summary of “Artificial Intelligence Research: A Review on Dominant Themes, Methods, Frameworks, and Future Research Directions”
We All See the News: AI is Here to Stay and is Evolving Quickly!
Technology is undoubtedly changing quickly, and Artificial Intelligence (AI) has become a pivotal force for innovation, revolutionizing sectors and fundamentally changing how we live day-to-day. The year 2024 serves as evidence of the remarkable progress achieved in AI, with data and developments illustrating its extensive integration and influence. But all the hype and AI buzzwords in the News and social media can be confusing for those not well-versed in what AI is and what it can do. And, this uncertainty can create some anxieties for businesses and individuals alike. Even for the “average citizen”, understanding advancements in AI and being able to separate facts from myths is important to understand. And understanding, all starts with awareness, reflection, and education.
AI Uncertainty from Business and Individuals
In a November 21st, 2023 article, “What the data says about Americans’ views of artificial intelligence” by Michelle Faverio and Alec Tyson, the authors share that according to surveys conducted by the Pew Research Center, a mounting wariness among Americans regarding the expanding influence of artificial intelligence (AI) in their everyday routines. Currently, 52% of Americans harbor more apprehension than enthusiasm about AI’s incorporation, with just 10% showing greater excitement than apprehension. Furthermore, 36% of participants acknowledge experiencing a blend of both excitement and concern regarding AI’s prevalence. Additionally, National University in their “131 AI Statistics and Trends for (2024)” states that “88% of non-users are unclear how generative AI will impact their life”. This wariness and uncertainty surrounding the expanding influence of artificial intelligence (AI) stems from several factors. As a result, businesses and individuals alike are grappling with the complexities of navigating an increasingly AI-driven world, unsure of its implications for their livelihoods, privacy, and overall well-being. But, as mentioned above, understanding starts with awareness, reflection, and education.
Therefore, let’s continue.
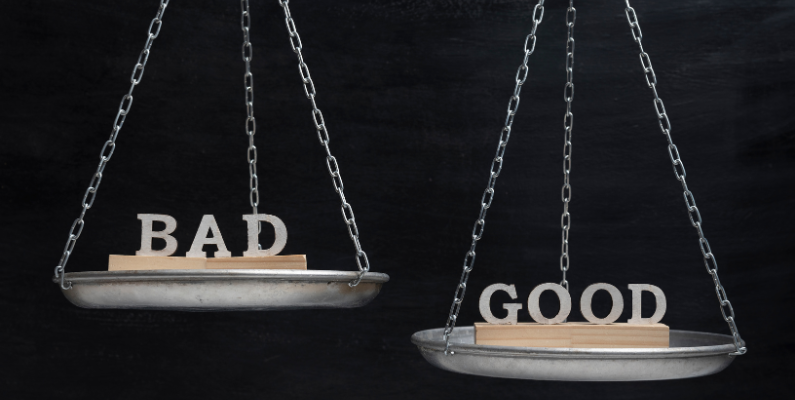
AI will bring change, negative and positive.
No Doubt About It: AI Will Bring Change
AI will bring change, negative and positive, but what are some of the negatives that people may be concerned about? Here is an outline of some common concerns:
- Job Displacement: Many people are concerned that AI and automation will lead to job loss or displacement, particularly in sectors where routine tasks can be easily automated, such as manufacturing, transportation, and customer service.
- Ethical Concerns: No doubt, there are ethical considerations surrounding the use of AI, including issues related to bias, privacy, surveillance, and potential misuse of AI technologies for harmful purposes.
- Loss of Control: There’s a fear of relinquishing control to machines, especially as AI systems become more autonomous and capable of making decisions that can significantly impact human lives.
- Economic Inequality: Concerns arise about the exacerbation of economic inequality, as those who have access to and control over AI technologies may benefit disproportionately compared to those who do not.
- Safety and Security: Worries about the safety and security implications of AI, including the potential for AI systems to malfunction or be hacked, leading to unintended consequences or malicious actions.
- Existential Risks: Some individuals and organizations express concerns about the long-term existential risks associated with the development of superintelligent AI, including scenarios where AI systems may surpass human intelligence and become difficult to control. We will discuss more about this point in upcoming articles and podcasts. (In the meantime, here is an interesting read: Landgrebe, J., & Smith, B. (2022). “Why machines will never rule the world: artificial intelligence without fear”.
We All Have a Role to Play in Determining the Future Role of AI
- Ethical Considerations: As AI technologies become more pervasive, ethical considerations become increasingly important. Each individual’s values and ethical judgments contribute to shaping the ethical frameworks that guide AI development and deployment.
- Regulatory Frameworks: Policymakers rely on input from various stakeholders, including individuals, to shape regulations around AI. These regulations determine how AI systems are developed, deployed, and used in various contexts, from healthcare to autonomous vehicles.
- Public Perception and Acceptance: Public perception and acceptance of AI technologies influence their adoption and implementation. Individuals’ attitudes, beliefs, and preferences shape how AI technologies are received and used in society.
- Skills and Education: Developing AI technologies requires a diverse range of skills and expertise. Individuals can contribute to the advancement of AI by pursuing education and training in relevant fields, such as computer science, data science, and ethics.
- Feedback and Iteration: Feedback from users and stakeholders is crucial for improving AI systems. By providing feedback on AI technologies, individuals can help identify shortcomings, biases, and areas for improvement, ultimately shaping the development of more effective and responsible AI systems.
- Social Impact: AI technologies have the potential to significantly impact society, including employment, healthcare, education, and more. Individuals can advocate for the responsible development and deployment of AI to ensure that its benefits are maximized while minimizing potential negative consequences.
Each organization’s and individual’s actions, choices, and contributions play a collective role in determining the future role of AI, influencing its development, regulation, acceptance, and societal impact. By actively engaging with AI technologies and participating in discussions surrounding their use, individuals can help shape a future in which AI serves the common good and aligns with human values and priorities.
How Can AI Research Help Address Future Uncertainty of AI deployment?
An understanding of AI, through objective, peer-reviewed, and collaborative AI research, can play a significant role in addressing the future uncertainty of AI deployment in several ways including advocating for engagement of more fields of study and research to drive policy. Determining AI policy involves a multidisciplinary approach, drawing insights from various fields of study to address the complex ethical, legal, social, economic, and technical considerations surrounding artificial intelligence. Here are some key fields that should engage in shaping AI policy:
-
- Computer Science and Engineering: Fundamental understanding of AI technologies, algorithms, and systems is crucial for crafting informed policies.
- Ethics and Philosophy: Discussions on the ethical implications of AI, including issues such as bias, fairness, accountability, transparency, and the impact on human rights and dignity.
- Law and Policy Studies: Legal frameworks and regulations concerning AI, including intellectual property, privacy, liability, and data protection laws, as well as international agreements and standards.
- Social Sciences: Societal impacts of AI, including its effects on employment, inequality, social cohesion, education, healthcare, and governance.
- Economics: Analysis of the economic implications of AI adoption, including productivity gains, job displacement, market competition, and redistribution of wealth.
- Psychology and Cognitive Science: Understanding human cognition and behavior in the context of AI interaction, including user trust, acceptance, and the psychological effects of automation.
- Cybersecurity: Assessing AI-related cybersecurity risks, such as vulnerabilities in AI systems, adversarial attacks, data breaches, and the potential for misuse by malicious actors.
- Environmental Science: Evaluating the environmental impact of AI technologies, including energy consumption, resource usage, and waste generation.
- International Relations and Geopolitics: Addressing geopolitical implications of AI development, including issues related to national security, global competition, arms control, and diplomacy.
- Bioethics and Health Policy: Examining ethical considerations in AI applications within healthcare, including patient privacy, consent, medical decision-making, and the potential for bias in healthcare algorithms.
- Education and Workforce Development: Identifying skill gaps, educational needs, and training opportunities related to AI technologies, as well as strategies for ensuring a resilient workforce in the age of automation.
- Urban Planning and Transportation: Integrating AI technologies into urban infrastructure and transportation systems, considering issues such as traffic management, sustainability, accessibility, and urban design.
- Human Rights and Social Justice: Ensuring that AI policies promote equality, diversity, inclusion, and respect for human rights, addressing concerns related to discrimination, surveillance, and the digital divide.
- Risk Management and Decision Theory: Assessing and managing risks associated with AI deployment, including uncertainty, unintended consequences, and the trade-offs between risks and benefits.
- Engagement from these above diverse fields is essential for developing comprehensive AI policies that balance innovation with societal values and address the complex challenges posed by AI technologies and to address and improve key areas such as below:
- Robustness and Reliability: AI researchers can focus on developing algorithms and models that are robust and reliable across different environments, datasets, and scenarios. By ensuring that AI systems perform consistently and accurately, even in unpredictable conditions, researchers can mitigate the uncertainty associated with AI deployment.
- Ethical and Responsible AI: Research in AI ethics and responsible AI can help establish guidelines and frameworks for the ethical deployment of AI systems. This includes addressing biases, fairness, transparency, and accountability in AI algorithms and decision-making processes, which can help alleviate concerns about the potential negative impacts of AI deployment.
- Interpretability and Explainability: Advancements in interpretable and explainable AI techniques enable users to understand and trust AI systems better. By making AI algorithms and models more transparent and interpretable, researchers can help stakeholders, including policymakers, regulators, and the general public, to better understand AI decisions and predictions, reducing uncertainty about AI deployment.
- Risk Assessment and Management: AI research can contribute to developing methods for assessing and managing risks associated with AI deployment. This includes identifying potential risks such as cybersecurity threats, unintended consequences, and societal impacts, and developing strategies to mitigate these risks effectively.
- Human-AI Collaboration: Research in human-AI interaction and collaboration can enhance the synergy between AI systems and human users, enabling more effective and trustworthy AI deployment. By designing AI systems that complement human strengths and mitigate human weaknesses, researchers can foster collaboration and cooperation between humans and AI, reducing uncertainty about the roles and impacts of AI in various domains.
- Adaptability and Resilience: AI research can focus on developing adaptive and resilient AI systems capable of learning and evolving over time. By designing AI algorithms that can continuously adapt to changing environments and requirements, researchers can help mitigate the uncertainty associated with future developments and challenges in AI deployment.
- Regulatory and Policy Frameworks: AI research can inform the development of regulatory and policy frameworks to govern the deployment of AI systems. By providing insights into the technical capabilities and limitations of AI technologies, researchers can contribute to the formulation of regulations and policies that promote the responsible and beneficial use of AI while addressing concerns about uncertainty and risk.
AI research plays a crucial role in addressing the future uncertainty of AI deployment by advancing the state-of-the-art in AI technologies, promoting ethical and responsible AI practices, enhancing transparency and interpretability, managing risks, fostering human-AI collaboration, developing adaptive systems, and informing regulatory and policy decisions.
A Word on Research: Looking for Trends and Themes
What is the value and purpose of looking for trends and themes in academic research? Objective, peer-reviewed academic research has a significant role to play in the continuation and application of problem-solving to the practical world. Here are some key examples of why:
- Identifying Emerging Areas of Interest: Trends and themes help researchers identify emerging topics and areas of interest within their field. By analyzing patterns in research, scholars can pinpoint where new ideas and concepts are gaining traction, enabling them to stay current and potentially contribute to cutting-edge developments.
- Guiding Research Directions: Understanding trends and themes helps researchers decide where to focus their efforts. By identifying gaps in existing literature or areas where consensus is lacking, scholars can tailor their research questions and methodologies to address relevant issues and contribute meaningfully to the scholarly conversation.
- Informing Policy and Practice: Trends and themes in academic research often have implications for real-world policy and practice. Policymakers, practitioners, and industry professionals rely on scholarly insights to inform their decision-making processes. By identifying trends and themes, researchers can offer evidence-based recommendations that have the potential to influence policies and practices in various domains.
- Facilitating Collaboration: Recognizing common themes and trends in research can facilitate collaboration among researchers working on similar topics. By connecting with others who share their interests, scholars can exchange ideas, share resources, and collaborate on projects, leading to more robust and impactful research outcomes.
- Enhancing Understanding: Analyzing trends and themes allows scholars to gain deeper understanding of the evolution of ideas within their field. By tracing the development of concepts and theories over time, researchers can uncover underlying patterns and relationships, shedding light on the broader intellectual landscape and fostering critical insights.
Looking for trends and themes in academic research not only helps researchers stay informed and relevant but also contributes to the advancement of knowledge within their discipline and beyond. And, ultimately to the world where it’s application can address real problems.
What is Artificial Intelligence (AI)?
“While AI is not entirely novel, its commercialization gained momentum around 2000. AI, in essence, is a simulation of human intelligence in computer systems [1]. AI comprises the development of algorithms, software, and hardware that enable machines to perform tasks that typically require human intelligence [2–4].” (Ofosu-Ampong, 2024).
Ofosu-Ampong continues:
“The AI phenomenon has its genesis in other technologies, namely computing power, machine learning, big data, cloud computing, opensource software, algorithms and virtualization [17,18]. Features that distinguish AI from related technologies are its ability to learn, adapt, reason and decision-making. In addition to handling uncertainty and noise, interaction and collaboration, and representation and abstraction [19,20]. Currently, there is no standard”
What Are Information Systems?
Information systems (IS) refer to the combination of hardware, software, data, people, and procedures that work together to collect, process, store, and distribute information within an organization. These systems play a crucial role in facilitating decision-making, coordinating processes, and achieving organizational goals efficiently and effectively. Information systems can vary widely in scope and complexity, ranging from simple systems like a spreadsheet application used for budgeting to complex enterprise-wide systems like Customer Relationship Management (CRM) or Enterprise Resource Planning (ERP) systems. Key components of information systems would include:
- Hardware: This includes computers, servers, networking equipment, and other physical devices necessary for processing and storing data.
- Software: Information systems rely on software applications to perform specific tasks, such as word processing, database management, and enterprise resource planning.
- Data: Data is the raw material that information systems process, store, and transmit. It can include text, numbers, images, and multimedia files.
- People: People are essential components of information systems, as they use the systems to input, manipulate, and interpret data. They also design, develop, maintain, and support the systems.
- Procedures: Procedures define how tasks are performed within an information system. They include rules, policies, workflows, and guidelines that govern the use and management of the system.
Information systems can serve various purposes within an organization, such as:
- Operational Support: Information systems automate routine tasks and support day-to-day operations, such as order processing, inventory management, and payroll processing.
- Decision Support: Information systems provide data and analytical tools to support decision-making at different levels of the organization, from strategic planning to tactical decision-making.
- Strategic Support: Information systems can help organizations gain a competitive advantage by providing insights into market trends, customer behavior, and industry dynamics.
Information systems are critical assets for modern organizations, enabling them to streamline processes, improve productivity, and adapt to changing business environments.
Ofosu-Ampong (2024): Dominant Themes
OK, still with me?
Now that we have laid some foundation, let’s dive into what Ofosu-Ampong shares as some common themes of his work. As Ofosu-Ampong describes, the principal themes are categorized to reflect the current progress in AI. The distribution of articles categorized under various AI research themes is outlined as follows: Technological issues constitute 36.5%, contextual issues account for 25.9%, domain and application make up 23.5%, and AI conceptualization represents 12.9%. Given that AI relies heavily on technical components such as machine learning, deep learning, NLP, speech recognition, fuzzy logic, and expert systems, it is unsurprising that the majority of literature falls under the technological issues theme. Ofosu-Ampong adds that this predominance also emphasizes the relatively lesser attention given to other themes, including AI contextual issues, conceptualization, and application domains.
The Technical or Technological Theme:
“There are growing concerns about the handling of sensitive data in AI systems due to potential misuse and breaches. The prevalence of AI systems is raising more concerns with regulatory frameworks and ethics.” (Ofosu-Ampong, 2024)
The technical theme revolves around the infrastructure facilitating data quality and accessibility. Within this research, various issues are explored, such as AI scalability, explainable AI, security, robustness, architecture, and interpretability. Studies on AI data quality and scalability illuminate the models and data supporting model development, advocating for enhancements in identifying and elucidating data errors. Furthermore, these investigations underscore the constant demand for vast quantities of high-quality data to enable AI to learn and generate accurate predictions, free from bias and inconsistencies that may yield inaccurate outcomes. Hence, enhancing AI performance hinges significantly on leveraging high-quality data across the four dimensions of volume, velocity, veracity, and variety. (Ofosu-Ampong, 2024)
Future research direction:
“Required to explore the mediating role of AI’s impact on performance e.g. academic or firm performance”. (Ofosu-Ampong, 2024). This would include how human-AI integration influences human-to-human interaction and on robust security measures and transparency.
The Contextual Theme:
“Contextually, AI applications can learn unintended and unforeseen behaviors from unstructured and complex data. This can lead to harmful or unintended consequences in societies.” (Ofosu-Ampong, 2024)
The theme of contextual knowledge in AI research encompasses socio-technical aspects such as acceptance, adoption, integration, cost, and ethics. Studies within this theme focus on collaboratively building context-specific knowledge to understand issues comprehensively. Factors influencing acceptance and adoption include psychological anthropomorphism, perceived value, technology readiness, management support, compatibility, and generalization of AI. Hindrances to adoption include privacy concerns, lack of education and training, transparency, trust, and cultural and social factors in some developing economies. Studies highlight concerns about privacy, trust, bias, fairness, regulatory frameworks, and legal challenges. The absence of universal laws governing AI adoption across sectors remains a significant challenge. Ensuring confidentiality and transparency, maintaining AI sustainability, and addressing cost concerns are also areas of focus. (Ofosu-Ampong, 2024
Future research direction:
“Investigate the sustainability of AI per context and compare results, should verify AI value and user retention via context and how do we combine different perspectives to build trust and collaboration with AI” (Ofosu-Ampong, 2024).
The Conceptualization Theme:
“AI models can act as “black boxes” making it difficult to understand its conceptualization and decision-making processes.” (Ofosu-Ampong, 2024)
The third major theme presented by Ofosu-Ampong is the conceptualization of artificial intelligence (AI). Several articles examine this domain, aiming to establish a foundation and entry point for AI. Given the early stage of AI development, it’s crucial to understand fundamental concepts and processes to advance the field. This theme examines AI research through three primary perspectives: operational, technical, and theoretical understanding. (Ofosu-Ampong, 2024
- Operational articles focus on elucidating how individuals grasp the practical aspects of AI and its potential deployment.
- Technical understanding involves a deeper exploration of the technical foundations of AI, including models, algorithms, and data processing techniques.
- Theoretical understanding of AI encompasses cognitive science, mathematical principles, and the philosophical underpinnings of intelligence and AI. This sub-theme poses profound questions about the nature of intelligence and how AI applications can emulate it.
Future research direction:
“Test new research frameworks and also explore integrative approaches to develop new ones to enhance our understanding of AI’s future directions.” (Ofosu-Ampong, 2024)
The Application Domain Theme:
“AI applications and data required have the potential to grow. However, there are issues with data storage, processing and analysis of infrastructure needed to manage the growth of AI (i.e. the domain of AI is showing an ever-increasing flow).” (Ofosu-Ampong, 2024)
The realm of AI application spans various fields, as evidenced by recent publications. These include scientific research, medicine, education, e-governance, legal services, industry, customer service, advisory systems, sustainable entrepreneurship, and computer network security. These studies highlight the positive impacts of AI across these domains and advocate for further development and utilization of AI-based systems to streamline processes. Scholars emphasize the need for expanding AI applications into less explored areas such as transportation, green IT, agriculture, climate modeling, cybersecurity, energy, utilities, compliance, and retail/e-commerce, where potential benefits remain untapped. (Ofosu-Ampong, 2024)
Future research direction:
“Venture into new unexplored domains like sustainability and green IT, Future research is required to advance our knowledge of how domain models differ from AI tailored solutions.” (Ofosu-Ampong, 2024)
Ofosu-Ampong’s Recommendations and AI Solutions for Businesses
This article shared the analysis of 85 academic articles to assess the prevailing issues and knowledge stock within artificial intelligence literature. Please read the full article here: “Artificial Intelligence Research: A Review on Dominant Themes, Methods, Frameworks and Future Research Directions”.
This research highlights the significance of addressing infrastructure for data quality and availability, as well as socio-technical aspects such as acceptance, adoption, and ethical considerations. Key takeaways from the summary include heightened interest in security and ethics, emphasis on business model transformation and digital innovation, and the evolving landscape of AI strategy and alignment. Here is the word for word general recommendation for businesses (p. 8):
- “The potential of AI workforce disruption and ethical issues requires an improved learning outcome, increased efficiency and research advancement.”
- “Discussing the future of business operation’s best practices, challenges and opportunities.”
- “Embrace individual changes, analytics and learning preferences and value the role of humans.”
- “Investing, recruiting and retaining employees who can design, and model AI-tailored solutions to business goals.”
Summary of “Artificial Intelligence Research: A Review on Dominant Themes, Methods, Frameworks, and Future Research Directions”
This article shared and provided an overview of a research paper by Dr. Ofosu-Ampong titled “Artificial Intelligence Research: A Review on Dominant Themes, Methods, Frameworks, and Future Research Directions.” Ofosu-Ampong outlines the main themes explored in contemporary AI research (in the context of AI in Information Systems), including technological issues, contextual knowledge, conceptualization, and application domains. Ofosu-Ampong’s paper emphasizes the importance of understanding and addressing challenges in AI, such as data quality, acceptance, adoption, and ethical considerations. It also discusses potential future research directions and recommendations for businesses to navigate the evolving landscape of AI. Overall, the article underscores the significance of AI research in driving innovation and addressing societal concerns. Contact Nestell & Associates today to learn more about how we can support your organization in the AI journey,
Dr. Jack G. Nestell
How Can AI Integration Aid Your Business Processes and Decision-making?
Artificial intelligence is reshaping how we manage IT and ERP systems within Private Equity. At Nestell & Associates, our expertise lies in seamlessly integrating advanced AI technologies to enhance your investment strategies and operational efficiencies. Our team is ready to tailor our technology and ERP advisory services to your specific needs. Visit our website or contact us directly to learn more about how our services can drive significant value for your firm.
Article References
Ofosu-Ampong, K. (2024), “Artificial Intelligence Research: A Review on Dominant Themes, Methods, Frameworks and Future Research Directions”. Telematics and Informatics Reports, 100127. https://www.sciencedirect.com/science/article/pii/S2772503024000136
Related Articles:
Bias in Artificial Intelligence-Enabled ERP Software Customization: Say whaaat?!
The Future of ERP: Intrinsically Linked to the Evolution of Artificial Intelligence
Machine Learning in ERP: Envisioning the Future of Business Performance
Intelligent ERP Systems: Artificial Intelligence and Its Impact on ERP Organizational Change
A Letter TO: CxOs FROM: Your Upcoming ERP Project
Related Podcast Episodes from “TheERP Organizational Change Journal”
Episode 85: Harnessing AI for ERP Innovation: Balancing Growth, Ethics, and Governance
Episode 89: AI’s Role in ERP and Organizational Evolution
Episode 38: How Artificial Intelligence is Transforming the ERP Systems
Latest Podcast Episodes – “The ERP Organizational Change Journal”
Human Capital in PE-Backed Companies: Strategies for Leadership and Talent Management
Human Capital in PE-Backed Companies: Strategies for Leadership and Talent ManagementEpisode Overview - PE-backed Human Capital Strategies Today’s episode centers on the pivotal role of human capital in PE-backed companies, emphasizing how strategic leadership and...
ERP Organizational Change: Technology Strategy
ERP Organizational Change: Technology Strategy and Keys to SuccessEpisode Overview - Technology Strategy In general, at the highest level of categorization, there seems to be consensus on the importance of people and culture, informational technology, and project...
AI and Private Equity Synergy: Fueling Business Transformation
AI and Private Equity Synergy: Fueling Business TransformationEpisode Overview - AI in PE Strategies In this episode, we explore how AI and Private Equity synergies are fueling business transformations and reshaping investment strategies and operational efficiencies....
About Nestell & Associates
Where People, Processes, and Technology Align
Nestell & Associates specializes in providing M&A ERP and IT consulting services for private equity firms and their portfolio companies. We offer a range of vendor-neutral services to support all stages of the investment cycle.
Moreover, we know how to effectively minimize or eliminate the issues you experience during M&A. With Private Equity Technology Solutions as 100% of our business, we bring a unique approach to ERP that other firms can’t compete with.